Methods & Software
Physics-based tools
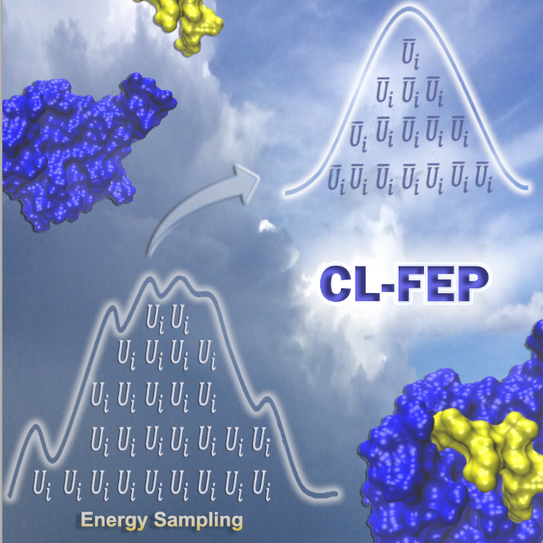
Central Limit Free Energy Perturbation (CL-FEP): a tool for accurate end–state free energy estimations
Binding free energy calculations are essential for the study of chemical and biological systems. However, accurate methodologies in the field are often computationally demanding, which limits their applicability. As an alternative, we introduced the Central Limit Free Energy Perturbation (CL-FEP) approach. Without computing intermediate points of a system transformation, CL-FEP can deliver accurate free energy change values. In CL-FEP, the exponential average estimator is evaluated upon transforming the energy output of molecular dynamics simulations by applying the Central Limit theorem. Importantly, energies from explicit solvent simulations can be used to evaluate the estimator and no fitted parameters are introduced in our implementation.
https://clfep.cbe.bci.tu-dortmund.de/
CL-FEP: an end-state free energy perturbation approach
Yasser B. Ruiz-Blanco and Elsa Sanchez-Garcia
Journal of Chemical Theory and Computation 2020, 16 (3), 1396-1410
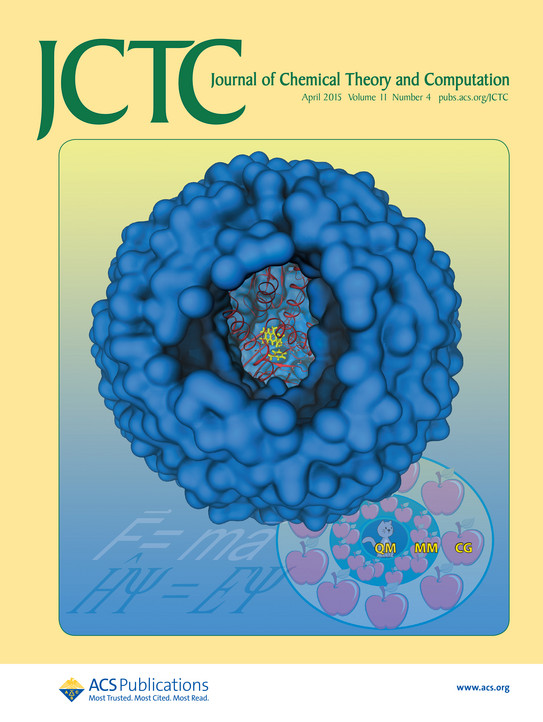
Multi-Scale Computational Approaches: QM/MM/CG implementation
Hybrid methods, typically Quantum Mechanics/Molecular Mechanics approaches (QM/MM), are at the core of our research. There is considerable interest in the development of coarse-grained (CG) force fields to improve the performance in MD simulations and geometry optimizations. We implemented a triple-layer Quantum Mechanics/Molecular Mechanics/Coarse Grained (QM/MM/CG) modelling approach to reduce computing times and extend the applicability of QM/MM calculations. We applied our implementation to the study of the effect of CG water on two enzymes: chorismate mutase and p-hydroxybenzoate hydroxylase.
Hybrid quantum mechanics/molecular mechanics/coarse grained modeling: A triple-resolution approach for biomolecular systems
Pandian Sokkar, Eliot Boulanger, Walter Thiel and Elsa Sanchez-Garcia
Journal of Chemical Theory and Computation 2015, 11 (4), 1809-1818
Quantum mechanics/molecular mechanics multiscale modeling of biomolecules
Joel Mieres-Perez and Elsa Sanchez-Garcia
Advances in Physical Organic Chemistry 2020, 54, 143-183
Machine learning-based tools
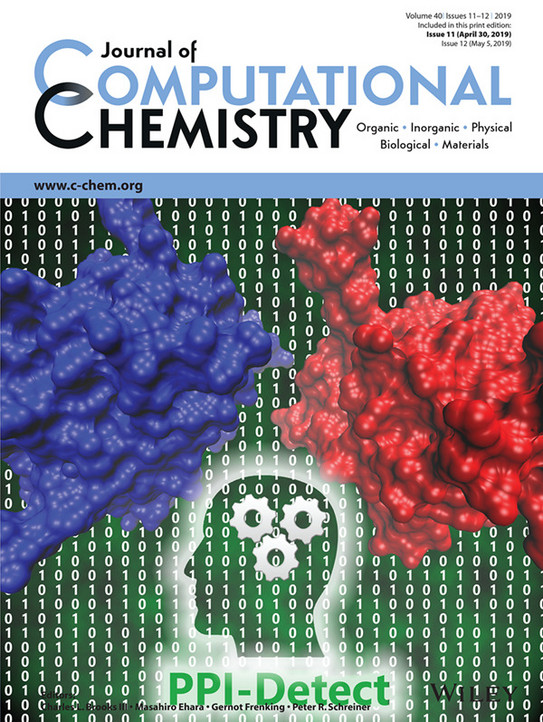
PPI-Detect: a tool for predicting the interaction likelihood of protein–protein and protein–peptide pairs
Machine learning methods allow exploiting the information content in protein datasets. We introduced a procedure for the general-purpose numerical codification of polypeptides. Using this codification, we developed a support vector machine model (PPI-Detect), which allows predicting whether two proteins will interact or not. PPI-Detect outperformed other state of the art sequence-based predictors of protein–protein interactions.
The PPI-Detect web server is available at the following link:
PPI‐Detect: A support vector machine model for sequence‐based prediction of protein–protein interactions
Sandra Romero‐Molina, Yasser B Ruiz‐Blanco, Mirja Harms, Jan Münch, Elsa Sanchez‐Garcia
Journal of Computational Chemistry 2019, 40 (11), 1233-1242
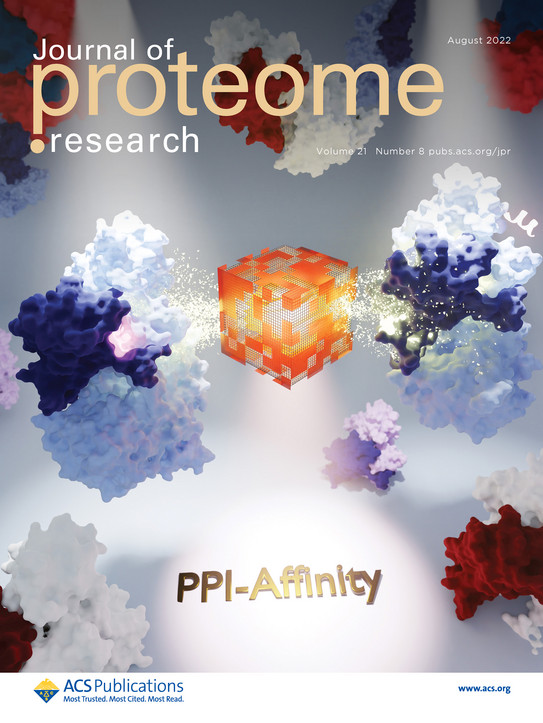
PPI-Affinity: a tool for predicting the binding affinity of protein–protein and protein–peptide complexes and for protein engineering applications
PPI-Affinity is a machine-learning tool developed to predict the binding affinity of protein–protein and protein–peptide complexes, based on their three-dimensional structure. We implemented PPI-Affinity as a web tool with three modules: (1) for estimating the binding affinity of protein – protein and protein – peptide complexes, (2) for estimating the affinity of specified mutants and as a (3) protein-engineering module for generating mutant complexes and estimating their affinity. PPI-Affinity thus facilitates the virtual screening of protein–protein and protein–peptide interactions, with direct applications for hit identification and optimization in drug design projects as well as for protein engineering.
The PPI-Affinity web server is available at the following link:
PPI-Affinity: A web tool for the prediction and optimization of protein–peptide and protein–protein binding affinity
Sandra Romero-Molina, Yasser B Ruiz-Blanco, Joel Mieres-Perez, Mirja Harms, Jan Münch, Michael Ehrmann and Elsa Sanchez-Garcia
Journal of Proteome Research 2022, 21 (8), 1829-1841
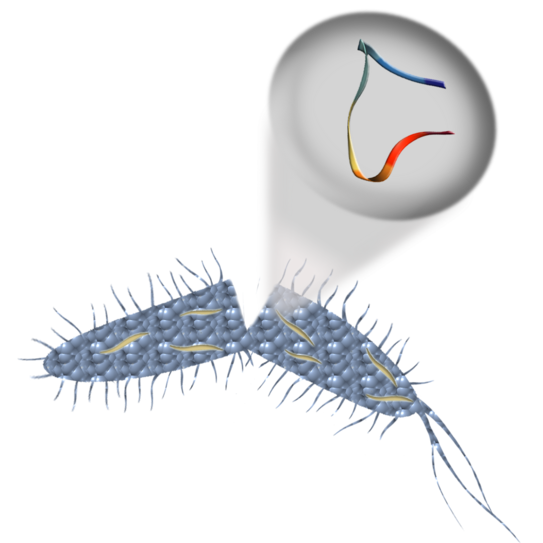
ABP-Finder: a machine-learning tool for the identification of antibacterial peptides
To address the problem of multi-drug resistance in bacteria, we developed ABP-Finder, a tool specifically designed to identify antibacterial peptides (ABPs) and for the estimation of which type of bacteria is targeted by these peptides. ABP-Finder ranks within the top state-of-the-art predictors of antibacterial peptides, particularly in terms of precision. We also applied ABP-Finder for the identification of an antibacterial peptide by screening the human urine peptidome.
The ABP-Finder web server is available at the following link:
ABP-Finder: A Tool to Identify Antibacterial Peptides and the Gram-Staining Type of Targeted Bacteria
Yasser B Ruiz-Blanco, Guillermin Agüero-Chapin, Sandra Romero-Molina, Agostinho Antunes, Lia-Raluca Olari, Barbara Spellerberg, Jan Münch and Elsa Sanchez-Garcia
Antibiotics (Special Issue Computational Approaches in Discovery & Design of Antimicrobial Peptides) 2022, 11 (12), 1708
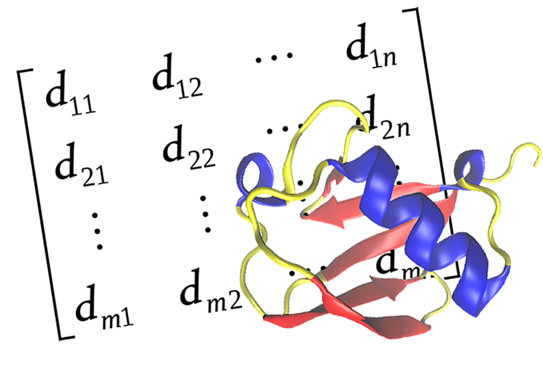
ProtDCal-Suite: a web server for studying proteins
We also introduced ProtDCal-Suite, a web server comprising a set of tools for studying proteins. The main module of ProtDCal-Suite is a software named ProtDCal (Ruiz-Blanco, Y.B., Paz, W., Green, J. et al. BMC Bioinformatics 16, 162 (2015). https://doi.org/10.1186/s12859-015-0586-0).
ProtDCal encodes the structural information of proteins in machine-learning-friendly vectors. Secondary modules of ProtDCal-Suite are tools developed for protein analysis that benefit from ProtDCal’s descriptors, including PPI-Detect, PPI-Affinity and ABP-Finder.
The ProtDcal suite web server is available at the following link:
ProtDCal‐Suite: A web server for the numerical codification and functional analysis of proteins
Sandra Romero‐Molina, Yasser B. Ruiz‐Blanco, James R. Green and Elsa Sanchez‐Garcia
Protein Science 2019, 28 (9), 1734-1743